Downloads
Download


This work is licensed under a Creative Commons Attribution 4.0 International License.
Review
Review of Digital Twin in the Automotive Industry on Products, Processes and Systems
Heli Liu 1,2, Benjamin Zhang 1, Vincent Wu 1,2, Xiao Yang 1,2, and Liliang Wang 1,2,*
1 Department of Mechanical Engineering, Imperial College London, London SW7 2AZ, UK
2 Smart Forming Research Base, Imperial College London, London SW7 2AZ, UK
* Correspondence: liliang.wang@imperial.ac.uk
Received: 17 February 2025; Revised: 10 March 2025; Accepted: 20 March 2025; Published: 24 March 2025
Abstract: In the era of digital manufacturing, digital technologies are rapidly revolutionising the automotive industry. Among these, the digital twin, an enabling industry 4.0 digital technology first introduced two decades ago, is characterised by the seamless integration of physical and cyber realms. The digital twin is undergoing extensive investigations within the automotive sector, covering various perspectives including design, manufacturing, and application. By leveraging the big manufacturing data captured by spatially distributed sensing networks, the digital twin shows the capacity to create high-fidelity models of actual manufacturing practices, thereby significantly improving the precision and efficiency of production processes. Integrated with other digital technologies such as big data analytics (BDA) and the Internet of Things (IoT), the digital twin mirrors components in the physical world into the virtual environment and facilitates the exchange of real-time information to achieve fully converged cyber-physical spaces. This in turn minimises costs and improves the overall product quality, flexibility of manufacturing processes, and system integration. This work reviewed recent advancements in digital twin applications in the automotive industry focusing on automotive products, manufacturing processes, and manufacturing systems. Insights were provided into the future of digitally enhanced technologies in automotive manufacturing towards digital manufacturing and developing digital product passports (DPPs) for circular economy (CE).
Keywords:
digital twin automotive industry 4.0 digitally enhanced technology digital product passports (DPPs) circular economy (CE)1. Introduction
In the digital manufacturing era, digital technologies are transforming various industries, including the automotive industry, at an unprecedented pace [1]. Representative promising strategies are Industry 4.0 (Germany) [2], Industrial Internet Consortium (USA) [3], Made in China 2025 (China) [4], and Society 5.0 (Japan) [5], coupled with the rapid advancement of cutting-edge digital technologies, including digital twin [6,7], digital product passports (DPPs) [8,9], Internet of Things (IoT) [10,11], Cyber-physical systems (CPS) [12–14], Artificial Intelligence (Al)-enhanced technologies and methods [15–17], big data analytics (BDA) [18–20] and cloud computing [21,22], are revolutionising the automotive industry at practically every aspect of the automotive sector, from the design and manufacturing to application phases. These technologies enable a more integrated and intelligent approach to facilitate enhanced efficiency, customisation, and innovation [23]. Empowered by these advanced digital tools, the automotive industry is experiencing significant enhancements in current manufacturing processes and paving the way for the next generation of automotive solutions, marking a significant advancement in the conception, production, and utilisation of vehicles.
The concept of the digital twin was first introduced in 2003 in a course entitled ‘Conceptual Ideal for Product Lifecycle Management (PLM)’ representing the linkage between real space and virtual space given by Prof. Michael Grieves from the University of Michigan [24]. National Aeronautics and Space Administration (NASA) unified the term ‘digital twin’ of this kind of concept in its ‘2010 technology roadmap’ [25]. For now, the digital twin has become one of the most promising technologies for realising Industry 4.0 in every manufacturing sector [26]. The global digital twin market, valued at USD 5 billion in 2020, is expected to surge to USD 86 billion by 2028, with a compound annual growth rate of 42.7% [27]. The past decades have seen significant advancements in computer technology, enabling the development of intricate virtual models of physical entities and their subsequent integration within systems engineering [28]. Leveraging these advancements, the digital twin mirrors the physical entity (e.g., a manufacturing process, or an entire life cycle of the manufactured product) through various lifecycle phases. Through the establishment of a sophisticated high-fidelity model as the virtual entity, big data information is captured via spatially distributed sensing networks and includes diverse dissemination of real-time information, decision-making algorithms and methodologies, functional modules, or information platforms for industrial activities [29]. This high-fidelity model may be used in various simulations using the real-time captured data which can lead to potential enhancement [30].
From the formation stage of the concept of a digital twin (year 2003 to year 2011) through to the incubation stage (year 2011 to year 2014) and now towards the rapid growth stage (year 2014 to the present), various interpretations and investigations of the digital twin exist within the academic communities [26]. Of these, some studies concentrate on simulations within the realm of digital twin research [31,32]. Other studies have explored a broader scope, encompassing physical, virtual, and internal connectivity elements, which are widely recognised as mainstream by the majority of researchers [6,33]. These digital twin elements are not isolated and closely interact with one another to achieve unified and comprehensive outcomes [34]. As shown in Figure 1, the digital twin concept can be characterised by five dimensions, which consist of the physical entity, virtual entity, service for physical and virtual entities, digital twin data, and connections of these parts [35].
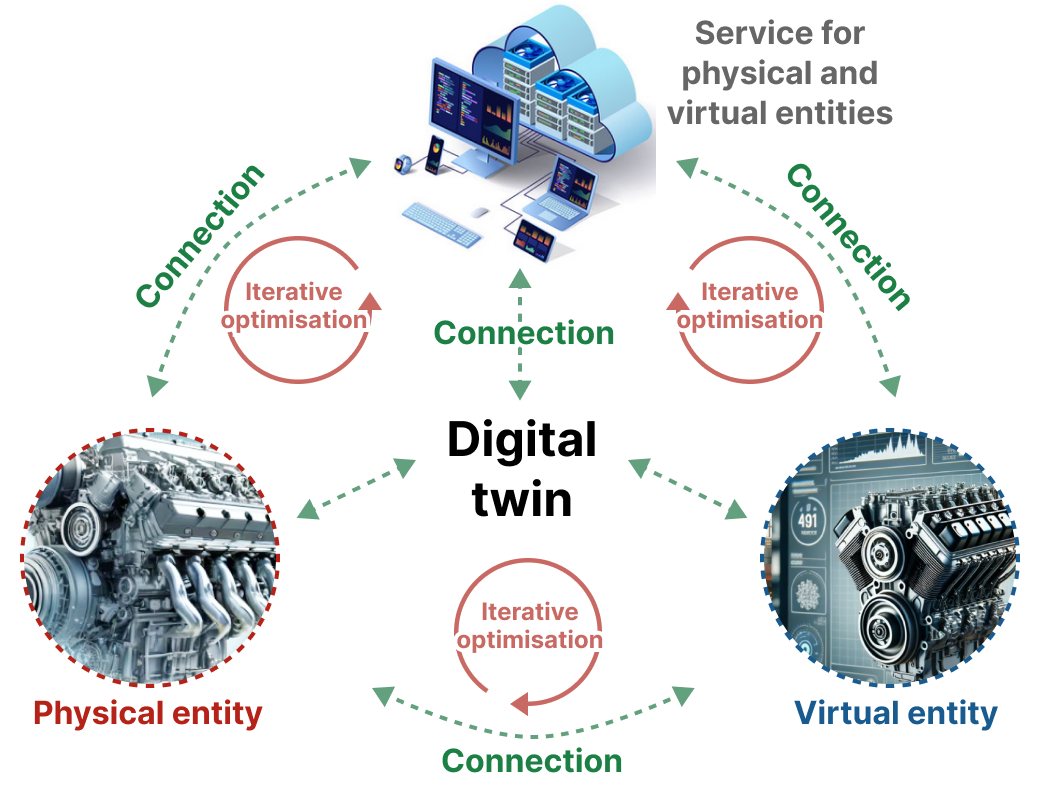
Figure 1. Five-dimensional demonstration of the concept of digital twin.
Figure 2 shows the key technologies and their corresponding domains for digital twins [36]. Therein, the technology architecture for digital twins is structured into three key domains: data-related technologies, high-fidelity modeling, and model-based simulation. Data-related technologies form the foundation by enabling data collection through Industrial IoT, sensors, RFID, cameras, and 3D scanning, while data mapping techniques such as XML, AutomationML, and OPC UA facilitate seamless communication. Data processing methods, including data fusion, blockchain, and edge computing, refine and enhance data quality, whereas data transmission technologies like Bluetooth, wireless sensor networks, and 5G ensure efficient connectivity. High-fidelity modeling serves as the core, incorporating physics-based models (e.g., geometrical, mechanical and material models), semantic modeling (e.g., machine learning, deep learning and expert systems), and model integration approaches such as flexible and multiphysics modeling to enhance accuracy and functionality. The model-based simulation plays a critical role in optimising performance by enabling multi-scale simulation, discrete event simulation, finite element analysis, exchange interfaces and bidirectional interaction, ensuring that digital twins accurately represent and predict real-world behaviours.
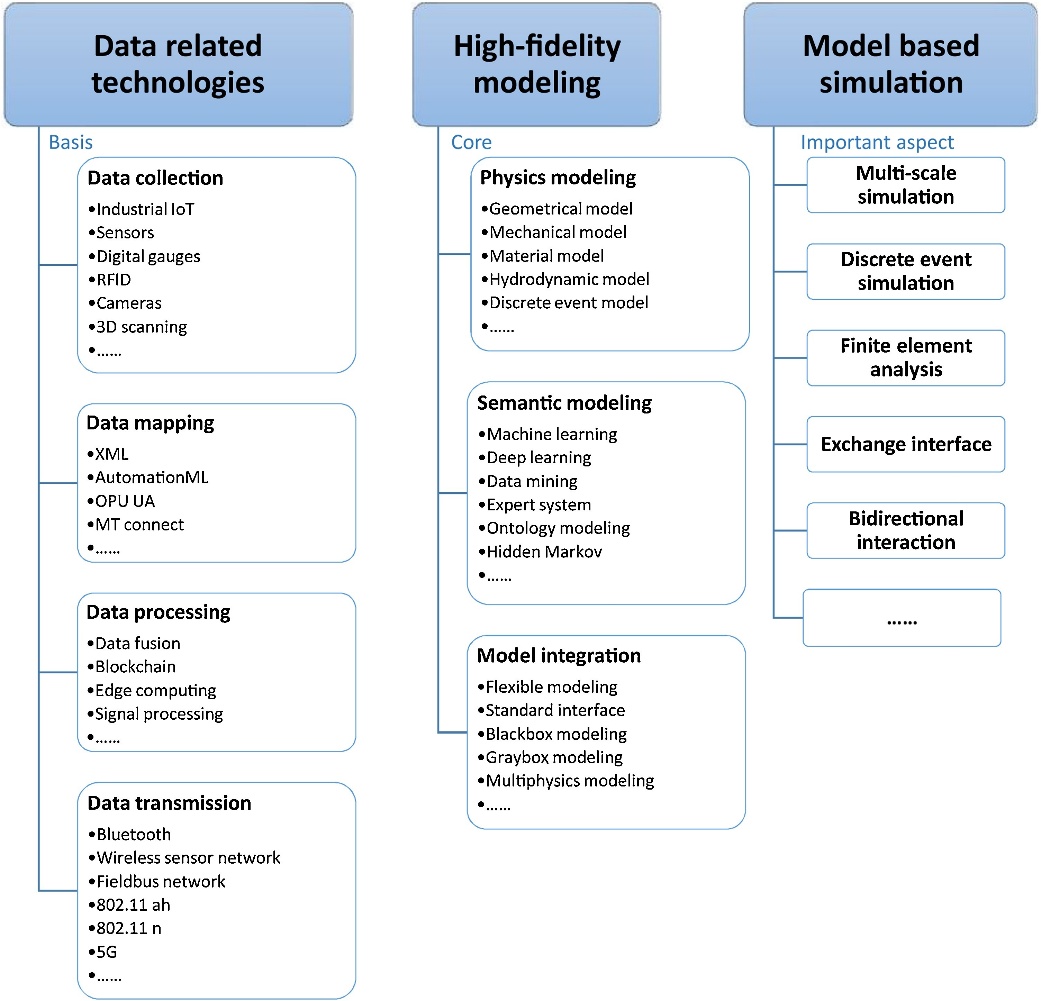
Figure 2. Digital twin key technologies and domains [36].
To date, few worldwide manufacturers have already explored integrating digital tools into their production workflows, which presents tremendous obstacles to realising the industry-scale transformation of digital manufacturing [37]. In this work, recent frontier studies—primarily from the past five years—were reviewed to examine the advancements of digital twins in the automotive industry, with a focus on their applications in varying levels of complexity addressed by automotive products, manufacturing processes, and manufacturing systems. In addition, perspectives were provided based on the review towards the future of digitally enhanced automotive manufacturing technologies towards digital manufacturing transformation.
2. Digital Twin in Automotive Products
In the rapidly evolving automotive industry, the advent of the digital twin demonstrates a significant advancement in automotive products [38], detailing their design, materials, components, functionality, and application. This approach enables the creation of a virtual replica of each automotive product or component in the physical world. This comprehensive physical-digital mapping facilitates real-time monitoring, analysis, and optimisation of vehicle performance under various environmental conditions (e.g., weather, temperature, and road condition) or different process parameters. The digital twin accelerates the product R&D phase and significantly reduces both the time and cost associated with the testing and validation of automotive components [39]. The adoption of a digital twin framework has created an adaptive and efficient development environment for automotive body control systems and their related components [40]. This strategy demonstrates exceptional adaptability, characterised by short development cycles, low complexity, reduced costs, enhanced scalability, and high flexibility. By mirroring real-world conditions in the virtual space, the digital twin facilitates a more responsive design process, leading to the optimised quality and performance of automotive products.
The Body in White (BIW) is a crucial component of the automotive manufacturing process, as it significantly influences the final appearance of a vehicle [41]. The digital twin was implemented into the design, pre-production, and production phases of the automotive BIW for real-time geometry assurance [42]. Figure 3 shows the digital twin input, activity, and final objective in this application. Specifically, in the design phase, finite element analysis (FEA) technology was embedded into the digital twin technology to conduct sensitivity and variation analyses for all sheet metal components. During the pre-production phase, the digital twin facilitated the update or generation of inspection features and inspection programs, and in the production phase, the digital twin enables the development of an assembly model for the automotive body informed by inspection data scanning the components geometries for adjusting locators and clamping positions [42].
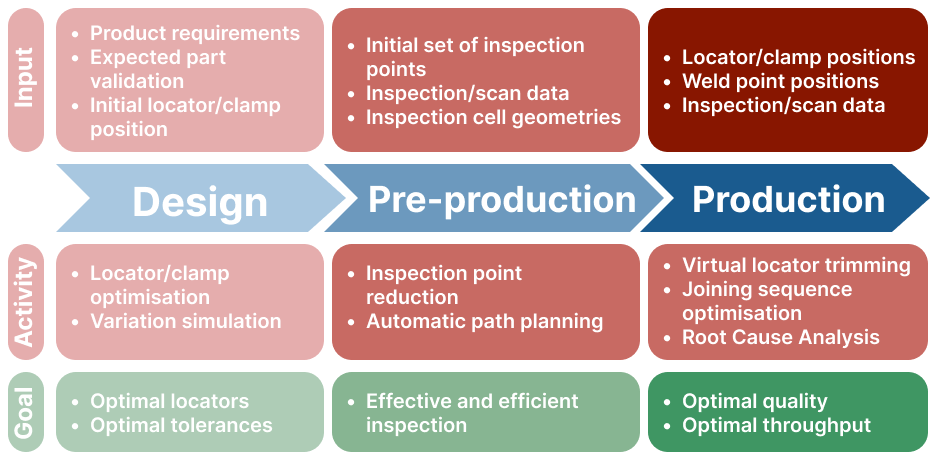
Figure 3. Digital twin input and functionality in the application of automotive component production [42].
While digital twins offer significant potential, they are not essential for every automotive product [30]. Not all products are complex enough to justify the extensive sensor networks and data flows required by digital twins. Additionally, from a financial perspective, investing substantial resources in developing a digital twin is not always cost-effective [30]. However, overall, digital twins are already used in many automotive industries, and the digital twin market in the automotive industry will continue to increase in the near future [43].
3. Digital Twin in Automotive Manufacturing Processes
In the context of processes, a digital twin focuses on the modelling of operations and workflow of the manufacturing process. Through simulating, analysing, and optimising the manufacturing process flow, identifying bottlenecks, and testing the impact of various conditions without disrupting the actual operations, the digital twin enhances the automotive manufacturing process including the blanking, pressing, and assembly processes for increasing efficiency, reducing costs, and improving product quality [44]. Various processes can be effectively modelled using different approaches, e.g., the automotive components forming process modelled using finite element (FE) simulation [45,46].
The utilisation of digital twin technology has significantly improved welding completeness in automotive BIW panels [47]. By adopting this approach, a mixed reality-oriented framework was developed specifically for the detection and configuration of welding completeness. Additionally, the widespread application of metal forming processes, such as the hot stamping process, significantly contributes to the manufacturing process of automotive parts like B-pillars and door inner panels. Advanced metal forming techniques, such as solution heat treatment, forming, in-die quenching (HFQ) [45,48], and fast light alloy stamping technology (FAST) [46,49,50], facilitate mass production of sheet components with high raw material usage and post-form material strength. In a case study, a digital twin-driven method was developed to monitor the tool wear behaviours in the stamping process [24]. Therein, the digital twin model consists of the punch force model, the forming quality model, and the model update strategy with consideration of the evolutionary tool wear. In addition, a digital twin-based stamping system was developed and verified in the incremental bending test with good accuracy and efficiency [51]. In a separate study, through the incorporation of deep learning algorithms, a digital twin was developed for the monitoring, control, and visualisation of welding joint growth, which significantly advances smart welding manufacturing [52]. This deep learning component substantially enhances the digital twin’s analytical capabilities, achieving a state-of-the-art accuracy in predicting the top-side bead width (TSBW) and back-side bead width (BSBW) from composite weld images.
A collaborative data management framework, enabled by digital twin technology, was conceptualised for the metal additive manufacturing (AM) process, a metal forming process gaining increasing attention due to superior design flexibility [53,54]. Figure 4 maps the number of published articles focusing on the essential elements of the AM digital twin in the past decade [55]. The data shows a significant rise in research publications over time, especially in higher-level digital twins (Levels 2 & 3), indicating a shift from basic modelling towards intelligent, adaptive systems with learning capabilities. The effectiveness of this framework was demonstrated through a cloud-based application scenario, which utilises deep learning for the analysis of layer defects in metal AM. The digital twin hierarchy was proposed for AM with four distinct tiers [55]. Level 0 is the Implicit digital twin, modelling a specific metal AM machine; Level 1 is the Instantiated digital twin which takes sensing technology as its interconnecting method; Level 2 is the Interfaced digital twin that enables the real-time monitoring, controlling and optimising; and Level 3, the Intelligent digital twin, which realises the sophisticated real-time decision making empowered by AI. This further emphasises the significance of digital twin technology in AM in developing high-fidelity, multiscale-multiphysics models, all of which are essential for enhancing the capabilities of AI in optimising AM processes [56]. Specifically, metal AM is a critical element of intelligent production systems and can be enhanced through the integration of AI-enhanced digital twins [57–59], which enables the optimisation of process parameters and topological designs [60]. In comparison, Level 0 digital twins focus primarily on static modelling, where physics-based simulations and computational models replicate the geometry and behaviour of a system without real-time data integration. These models are useful for initial design and testing but lack dynamic adaptation to operational conditions. In contrast, Level 3 digital twins incorporate intelligence and learning capabilities, enabling real-time data processing, predictive analytics, and autonomous decision-making. By integrating AI, machine learning, and advanced control mechanisms, Level 3 digital twins continuously refine the models based on live sensor data, optimising performance, detecting anomalies, and enabling self-improving systems [61]. This transition from passive representation (Level 0) to active intelligence (Level 3) enhances efficiency, reduces downtime, and supports adaptive manufacturing, making it a crucial advancement for future industrial applications.
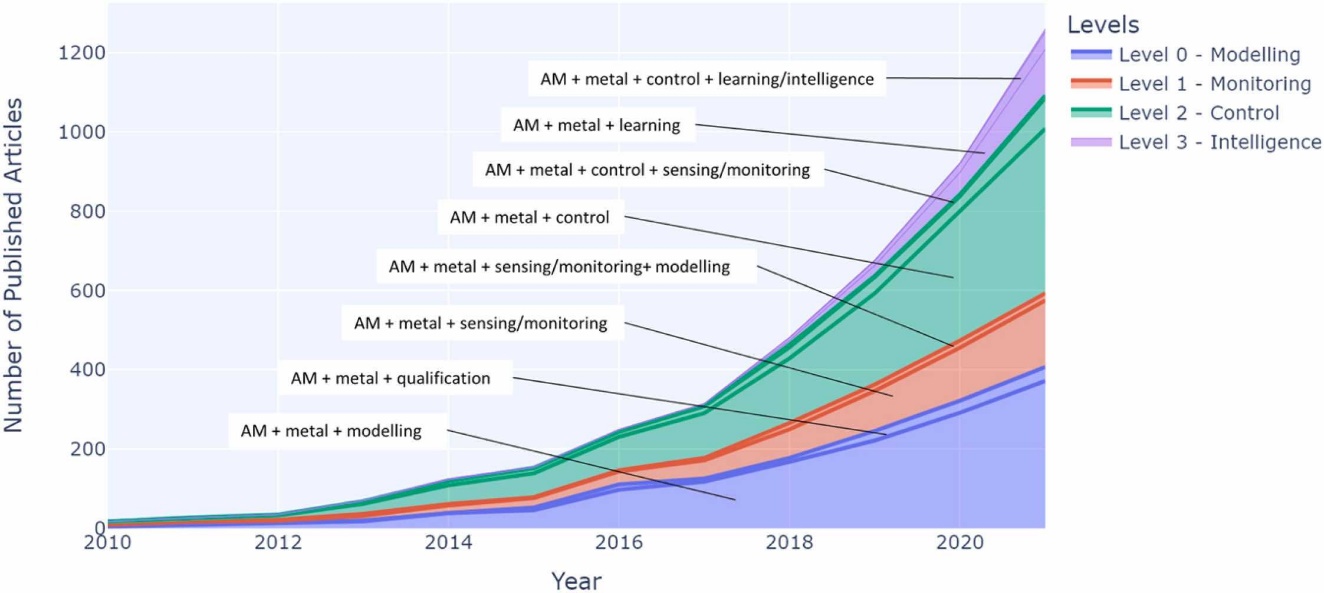
Figure 4. The number of published articles focusing on essential elements of the AM Digital Twin in the past decade [55].
4. Digital Twin in Automotive Manufacturing Systems
Encompassing a broader integration of multiple products and processes, the automotive manufacturing systems, such as the entire plant of an automotive manufacturer, are best represented in a virtual environment through the utilisation of digital twin for providing a holistic view of the system’s performance [62,63]. For instance, tailored for automotive body production lines, an integrated digital twin structure enabled immediate visualisation of daily production validations on a cloud platform, accelerating the decision-making process [64]. This technological framework enhanced operational and production prediction efficiency within the automotive body production lines. Another case study on digital twin was carried out in the commercial production phase of an automotive production line [65]. The digital twin architecture adopted consisted of several key modules including the data generation module enabled by sensing networks, data collection and distribution module, data visualisation module, data analysing module, and database communication module for interconnecting these elements via AI enhanced techniques. Results demonstrate a significant enhancement in operational efficiency, with a 6.01% increase in the commercial production line efficiency and an 87.56% gain in downtime.
The framework of digital twin-driven production line was designed for automotive micro-electro-mechanical system pressure sensors [66]. This framework encompasses key elements of a digital twin and ensures continuous, real-time data exchange between the physical production line and its digital counterpart, aiming to achieve digital manufacturing with minimal investment and labour costs. However, one of the considerable challenges presented is the development of prediction models and optimisation algorithms, driven by big data, to effectively manage and analyse a large array of process parameters. Additionally, a two-phase digital-twin-assisted fault diagnosis method was developed using deep transfer learning for a car body-side production line [67]. Therein, a virtual entity with virtual design and manufacturing platform was established, characterising manufacturing features, processes, resources and standards. The fault diagnosis model embedded in the digital twin was fully trained using the simulation data to improve prediction performance. Subsequently, the physical entity was established and connected to the virtual counterpart, and the DTL was used to generate a diagnosis model by transferring the knowledge obtained in previous phases.
A digital twin of the BIW production system was developed for initial and conceptual planning projects utilising current information from the CPS and a 3D scan [68]. Within this framework, the automated generation of a resource bill, facilitated by integrating data from robotic simulation and the CPS, significantly enhances the accuracy of digital twins in conceptual planning. Research within the automotive sector indicates a critical demand for digital twin technology in enhancing production and integration planning [69]. Consequently, employing digital twins for integration planning emerges as a pivotal area for future exploration in manufacturing settings. The escalating complexity, especially with the incorporation of electric vehicles, necessitates the creation of an automated method for generating digital twins to effectively address these challenges. In Figure 5, the components and functionalities of the digital twin ecosystem tailored for smart electric vehicles are showcased [63]. This includes a cloud-based interconnection framework that is augmented by technologies associated with physical entities, namely sensing, intelligent actuation, augmented reality, edge computing, and vehicle health monitoring, alongside virtual entity technologies such as AI, 3D CAD modeling, big data analytics, machine modeling, and predictive simulation.
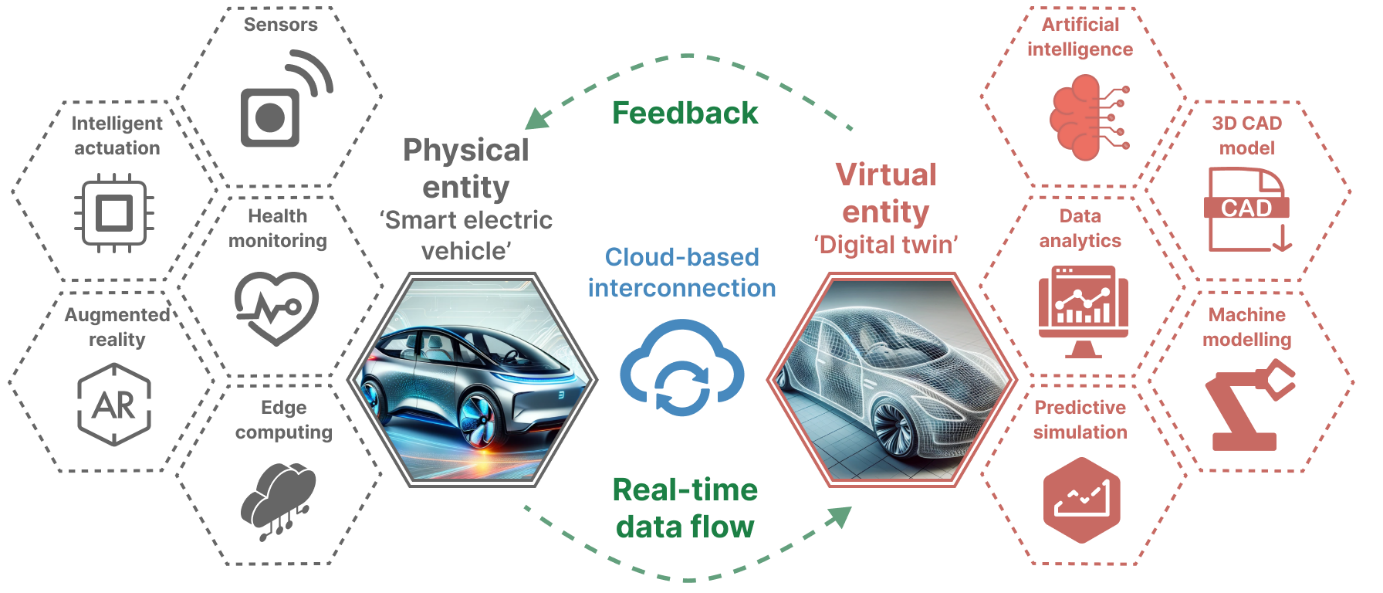
Figure 5. Ingredients and features of the digital twin ecosystem for smart electric vehicles [63].
5. Digital Twin towards Developing Digital Product Passports (DPPs) for Manufactured Products
The digital product passports (DPPs), promoted by the European Commission as a system of potential circular economy solutions [70], are unique product identifiers and serve as permanent traceable sources covering the entire life cycle of the products. DPPs act as potential enablers in the transition to more sustainable and circular production and consumption systems [71]. The DPPs highlight the importance of data, where three data tiers can be classified, i.e., data collection, data curation and sharing, and data leveraging [70]. In the automotive industry, integrating DPPs with digital twins provides real-time insights into a vehicle component, maintenance history, and sustainability metrics. This enhances predictive maintenance, regulatory compliance, and end-of-life planning, ensuring full lifecycle transparency. Aligned with the circular economy (CE), digital twins can optimise vehicle design for recyclability and remanufacturing, reducing waste and environmental impact. Manufacturers can leverage them to enhance resource efficiency and improve recycling processes, supporting sustainability in automotive production.
Similar to a CPS and an IoT, data collection in digital twin technology is enabled by the widespread installation of sensing networks, which is the mainstream data collection approach in Industry 4.0 [55]. The data flow should be steady and continuous [65]. Based on this, the complete data chain collected from a digital twin, across all stages of a product’s life cycle–from design and development to the end of life–enables the traceability of targeted products or services across various features including origins, materials, components and reusability, leading to the thorough data extraction for related DPPs [72]. Furthermore, data collection strategies can be progressively optimised through successive iterations, leveraging the insights derived from the available data collected by the digital twin [73]. This iterative optimisation refines the data collection policies to ensure maximum efficiency and relevance of the collected data.
AI-ML enhanced techniques are widely used in data curation such as data sorting and pre-processing [74]. Note that the manufacturing metadata collection from sensing networks is mostly likely to be fragmental data, consisting of a few (e.g., 1–2) information pieces, posing significant challenges to data curation and sharing. This phenomenon is very common in the manufacturing process of automotive components, as evidenced by the disrupted temperature readings of a workpiece. Imaging a factory equipped with 1,000 sensors transmitting signals at 10-s intervals, the data inflow can reach 360,000 entries per hour, which translates to over 8 million entries daily, significantly increasing the availability of fragmental data [75]. To address this issue, physics-informed data processing techniques are being employed by integrating fundamental physical principles into the analysis of data to enhance interpretability and general performance with a small volume of labelled data [76]. Furthermore, an efficient cloud-based platform is necessary for data curation and knowledge sharing following the well-known FAIR (Findable, Accessible, Interoperable, and Reusable) data principles [77-79]. For instance, a group of researchers pioneered the cloud-based FEA technologies that enable advanced multi-objective FEA of manufacturing processes that effectively bridge the gap between fundamental research and industrial applications [80–84]. Commercial cloud-based services, such as the AutoForm and ESI clouds, were developed to enhance computational efficiency. Unlike the traditional Software-as-a-Service (SaaS), this cloud-based platform is characterised by the framework of Model-as-a-Service (MaaS) for tackling future digital challenges. The platform is decentralised and encourages users to share and exchange frontier knowledge by generating ready-to-use models or scientific plugins, thus leading to tremendous CO2 emission reduction during the model R&D phase [84]. By using AI technologies, the uploaded models and published publications can be converted into applications that are compatible with the platform for global users. The effectiveness of these models, complied as ready-to-use functional modules, was successfully validated in peer-reviewed studies towards automotive applications [80,81,83,85].
Leveraging data obtained from digital twins assists the overall process enhancement of generating sustainable DPPs. As one of the data leveraging technologies, Blockchain technologies are increasingly used to guarantee the traceability and data privacy [86,87]. In addition, mapping the complete data chain collected from a digital twin enhances the traceability of the DPPs [88]. In this case, the digital characteristics (DC) were developed and defined as the visualisation of manufacturing metadata for a targeted manufacturing process incorporating essential information spanning the design, manufacturing, and application stages of manufactured products [89–91].
Furthermore, the DC space of manufacturing processes was developed by containing the most up-to-date and comprehensive manufacturing DC, as shown in Figure 6 [92,93]. Therein, each process can be accurately identified in the manufacturing DCS through calibrated angles (e.g., the forging process corresponds to an angle range of 21.97°–37.66°) or normalised values (e.g., the forging process falls within a range of 0.24–0.42) [92]. These distribution characteristics of the DC serve as valuable references, offering the potential to extract meaningful manufacturing insights even from fragmental data with extremely limited information [92]. By capturing the very unique digital features, the DC serves as the ‘DNA’ of any specific manufacturing process, thus the DCS serves as the ‘GenBank’ of the diverse manufacturing process [94-96]. The development of DC and DCS of manufacturing processes is expected to visualise the DPPs, and enables the assessment and verification of sustainability metrics, such as CO2 footprint and compliance with social standards, as well as support circular economy goals by minimising the CO2 footprint of targeted manufactured products.
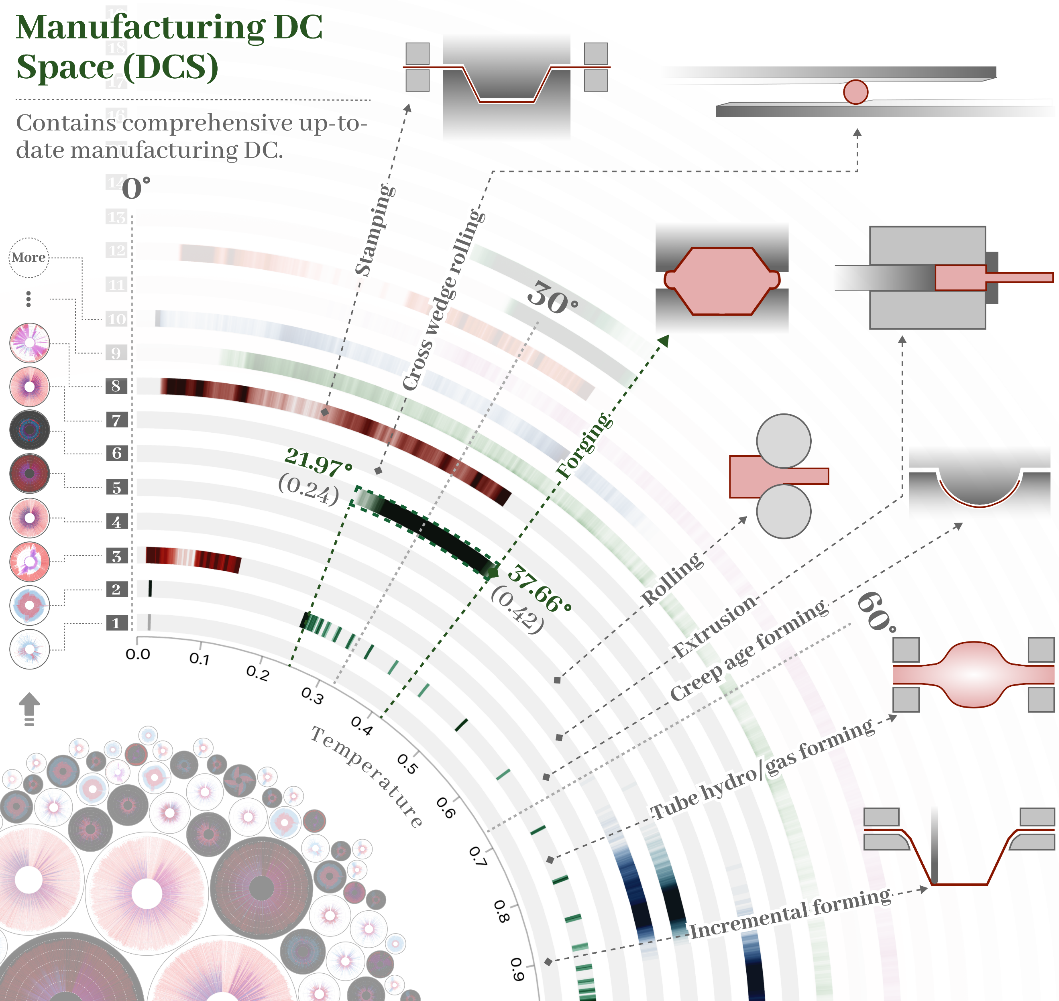
Figure 6. The concept of digital characteristics space (DCS) of manufacturing processes represented using temperature DC [92].
6. Discussion
The digital twin is rapidly transforming the automotive industry, serving as a key enabling technology for optimisation of product quality, enhancement of manufacturing process performance, and establishment of a digital framework for intelligent automotive manufacturing production lines and systems. Digital twins provide virtual models that offer dynamic replicas of physical assets, facilitating real-time monitoring, analysis, and simulation to drive efficiencies and innovations. Digital product passports (DPPs) can leverage the simulation data from digital twins to provide stakeholders with deeper insights into the product’s environmental impact, efficiency, and compliance with regulations. This relationship enhances the traceability and transparency of products throughout the entire life cycle, contributing to better-informed decision-making processes for manufacturers, consumers, and recyclers, leading to the realisation of circular economy (CE) objectives.
However, the application of digital twins faces several key challenges. Ensuring real-time data flow between physical robots and their digital twins is complex in the automotive industry. Digital twin simulations, especially in robotics, require massive computational power for real-time processing [97]. In some cases, automotive manufacturing still relies on older robotic platforms that were not specifically designed for digital twins. Furthermore, data availability surges during daily automotive production and variability in sensor precision can introduce data inconsistencies. Thus, data pre-processing procedures including data cleaning and sorting are crucial to ensure that high-quality data is fed into the virtual counterparts. This is essential to enhance the accuracy and reliability of simulations and predictions. As digital twin technology evolves, its integration with emerging technologies such as artificial intelligence and machine learning will further enhance its capabilities. The fusion with such technologies will address the challenge of processing vast volumes of data in real time. Meanwhile, the increasing demand for computational resources, data storage, and real-time data processing capabilities necessitates substantial upgrades to existing IT frameworks. Ensuring robust, scalable, and secure IT infrastructure is essential to fully unlock the potential of digital twins.
Despite these challenges, a crucial application of digital twins lies in smart manufacturing and supply chain optimisation. By connecting real-time data from production lines with digital replicas, manufacturers can achieve greater operational efficiency through adaptive process control and automated decision-making. This level of connectivity supports agile and resilient supply chains, enabling rapid adjustments to disruptions, such as material shortages or demand fluctuations. Furthermore, in the long term, the convergence of digital twins, artificial intelligence (AI), and IoT will unlock unprecedented possibilities. AI-driven digital twins will facilitate autonomous decision-making, while DPPs will enhance traceability and accountability throughout the supply chain. These advancements will drive the automotive industry toward greater sustainability, efficiency and innovation, marking a new era of intelligent manufacturing and lifecycle management.
Author Contributions: H.L.: conceptualization, methodology, visualization, writing—original draft preparation; B.Z.: investigation, writing—original draft preparation; V.W.: investigation, writing—reviewing and editing; X.Y.: writing—reviewing and editing; L.W.: supervision. All authors have read and agreed to the published version of the manuscript.
Funding: This research received no external funding.
Institutional Review Board Statement: Not applicable.
Informed Consent Statement: Not applicable.
Data Availability Statement: Not applicable.
Conflicts of Interest: The authors declare no conflict of interest.
References
- Liu, H.; Saksham, D.; Shen, M.; Chen, K.; Wu, V.; Wang, L. Industry 4.0 in metal forming industry towards automotive applications: A review. Int. J. Automot. Manuf. Mater. 2022, 1, 2. https://doi.org/10.53941/ijamm0101002.
- Sepasgozar, S.M.E.; Shi, A.; Yang, L.; Shirowzhan, S.; Edwards, D.J. Additive Manufacturing Applications for Industry 4.0: A Systematic Critical Review. Buildings 2020, 10, 231. https://doi.org/10.3390/buildings10120231.
- Weber, P.; Hiller, S.; Lasi, H. Design and Evaluation of an Approach to Generate Cross-Domain Value Scenarios in the Context of the Industrial Internet of Things: A Capability-Based Approach. In Proceedings of the 2019 Portland International Conference on Management of Engineering and Technology (PICMET), Portland, OR, USA, 25–29 August 2019; pp. 1–8. https://doi.org/10.23919/PICMET.2019.8893687.
- Li, L. China’s manufacturing locus in 2025: With a comparison of “Made-in-China 2025” and “Industry 4.0”. Technol. Forecast. Soc. Change 2018, 135, 66–74. https://doi.org/10.1016/j.techfore.2017.05.028.
- Aquilani, B.; Piccarozzi, M.; Abbate, T.; Codini, A. The Role of Open Innovation and Value Co-creation in the Challenging Transition from Industry 4.0 to Society 5.0: Toward a Theoretical Framework. Sustainability 2020, 12, 8943. https://doi.org/10.3390/su12218943.
- Tao, F.; Qi, Q. Make more digital twins. Nature 2019, 573, 490–491. https://doi.org/10.1038/d41586-019-02849-1.
- VanDerHorn, E.; Mahadevan, S. Digital Twin: Generalization, characterization and implementation. Decis. Support Syst. 2021, 145, 113524. https://doi.org/10.1016/j.dss.2021.113524.
- Gligoric, N.; Krco, S.; Hakola, L.; Vehmas, K.; De, S.; Moessner, K.; Van Kranenburg, R. SmartTags: IoT Product Passport for Circular Economy Based on Printed Sensors and Unique Item-Level Identifiers. Sensors 2019, 19, 586. https://doi.org/10.3390/s19030586.
- Mulhall, D.; Ayed, A.-C.; Schroeder, J.; Hansen, K.; Wautelet, T. The Product Circularity Data Sheet—A Standardized Digital Fingerprint for Circular Economy Data about Products. Energies 2022, 15, 3397. https://doi.org/10.3390/en15093397.
- Haghnegahdar, L.; Joshi, S.S.; Dahotre, N.B. From IoT-based cloud manufacturing approach to intelligent additive manufacturing: Industrial Internet of Things—An overview. Int. J. Adv. Manuf. Technol. 2022, 119, 1461–1478. https://doi.org/10.1007/s00170-021-08436-x.
- Sisinni, E.; Saifullah, A.; Han, S.; Jennehag, U.; Gidlund, M. Industrial Internet of Things: Challenges, Opportunities, and Directions. IEEE Trans. Ind. Inform. 2018, 14, 4724–4734. https://doi.org/10.1109/TII.2018.2852491.
- Tao, F.; Qi, Q.; Wang, L.; Nee, A.Y.C. Digital Twins and Cyber–Physical Systems toward Smart Manufacturing and Industry 4.0: Correlation and Comparison. Engineering 2019, 5, 653–661. https://doi.org/10.1016/j.eng.2019.01.014.
- Leitão, P.; Colombo, A.W.; Karnouskos, S. Industrial automation based on cyber-physical systems technologies: Prototype implementations and challenges. Comput. Ind. 2016, 81, 11–25. https://doi.org/10.1016/j.compind.2015.08.004.
- Babiceanu, R.F.; Seker, R. Big Data and virtualization for manufacturing cyber-physical systems: A survey of the current status and future outlook. Comput. Ind. 2016, 81, 128–137. https://doi.org/10.1016/j.compind.2016.02.004.
- Bustillo, A.; Pimenov, D.Y.; Mia, M.; Kapłonek, W. Machine-learning for automatic prediction of flatness deviation considering the wear of the face mill teeth. J. Intell. Manuf. 2021, 32, 895–912. https://doi.org/10.1007/s10845-020-01645-3.
- Wuest, T.; Weimer, D.; Irgens, C.; Thoben, K.-D. Machine learning in manufacturing: Advantages, challenges, and applications. Prod. Manuf. Res. 2016, 4, 23–45. https://doi.org/10.1080/21693277.2016.1192517.
- Monostori, L. AI and machine learning techniques for managing complexity, changes and uncertainties in manufacturing. Eng. Appl. Artif. Intell. 2003, 16, 277–291. https://doi.org/10.1016/S0952-1976(03)00078-2.
- Kubik, C.; Knauer, S.M.; Groche, P. Smart sheet metal forming: Importance of data acquisition, preprocessing and transformation on the performance of a multiclass support vector machine for predicting wear states during blanking. J. Intell. Manuf. 2022, 33, 259–282. https://doi.org/10.1007/s10845-021-01789-w.
- Belhadi, A.; Zkik, K.; Cherrafi, A.; Yusof, S.M.; El fezazi, S. Understanding Big Data Analytics for Manufacturing Processes: Insights from Literature Review and Multiple Case Studies. Comput. Ind. Eng. 2019, 137, 106099. https://doi.org/10.1016/j.cie.2019.106099.
- Dai, H.-N.; Wang, H.; Xu, G.; Wan, J.; Imran, M. Big data analytics for manufacturing internet of things: Opportunities, challenges and enabling technologies. Enterp. Inf. Syst. 2020, 14, 1279–1303. https://doi.org/10.1080/17517575.2019.1633689.
- Helo, P.; Phuong, D.; Hao, Y. Cloud manufacturing–Scheduling as a service for sheet metal manufacturing. Comput. Oper. Res. 2019, 110, 208–219. https://doi.org/10.1016/j.cor.2018.06.002.
- Xu, X. From cloud computing to cloud manufacturing. Robot. Comput.-Integr. Manuf. 2012, 28, 75–86. https://doi.org/10.1016/j.rcim.2011.07.002.
- Zhong, R.Y.; Xu, X.; Klotz, E.; Newman, S.T. Intelligent Manufacturing in the Context of Industry 4.0: A Review. Engineering 2017, 3, 616–630. https://doi.org/10.1016/J.ENG.2017.05.015.
- Gan, L.; Li, L.; Huang, H. Digital Twin-Driven Sheet Metal Forming: Modeling and Application for Stamping Considering Mold Wear. J. Manuf. Sci. Eng. 2022, 144, 121003. https://doi.org/10.1115/1.4054902.
- Piascik, B.; Vickers, J.; Lowry, D.; Scotti, S.; Stewart, J.; Calomino, A. Materials, Structures, Mechanical Systems, and Manufacturing Roadmap. NASA. 2012. Available online: https://www.researchgate.net/profile/Rai-Waqas-Khan/post/can_you_share_the_manufacturing_process_and_materials_required_for_deployable_composite_boom_for_solar_arraysand_process_too/attachment/5a71d9dc4cde266d5887b8b1/AS%3A588877643395072%401517410779959/download/501625main_TA12-ID_rev6_NRC-wTASR.pdf (accessed on 27 February 2024).
- Tao, F.; Zhang, H.; Liu, A.; Nee, A.Y.C. Digital Twin in Industry: State-of-the-Art. IEEE Trans. Ind. Inform. 2019, 15, 2405–2415. https://doi.org/10.1109/TII.2018.2873186.
- Wang, Z.; Gupta, R.; Han, K.; Wang, H.; Ganlath, A.; Ammar, N.; Tiwari, P. Mobility Digital Twin: Concept, Architecture, Case Study, and Future Challenges. IEEE Internet Things J. 2022, 9, 17452–17467. https://doi.org/10.1109/JIOT.2022.3156028.
- Lu, S.C.-Y.; Li, D.; Cheng, J.; Wu, C.L. A Model Fusion Approach to Support Negotiations during Complex Engineering System Design. CIRP Ann. 1997, 46, 89–92. https://doi.org/10.1016/S0007-8506(07)60782-3.
- Uhlemann, T.H.-J.; Lehmann, C.; Steinhilper, R. The Digital Twin: Realizing the Cyber-Physical Production System for Industry 4.0′. Procedia CIRP 2017, 61, 335–340. https://doi.org/10.1016/j.procir.2016.11.152.
- Piromalis, D.; Kantaros, A. Digital Twins in the Automotive Industry: The Road toward Physical-Digital Convergence. Appl. Syst. Innov. 2022, 5, 65. https://doi.org/10.3390/asi5040065.
- Gabor, T.; Belzner, L.; Kiermeier, M.; Beck, M.T.; Neitz, A. A Simulation-Based Architecture for Smart Cyber-Physical Systems. In Proceedings of the 2016 IEEE International Conference on Autonomic Computing (ICAC), Wuerzburg, Germany, 17–22 July 2016; pp. 374–379. https://doi.org/10.1109/ICAC.2016.29.
- Weyer, S.; Meyer, T.; Ohmer, M.; Gorecky, D.; Zühlke, D. Future Modeling and Simulation of CPS-based Factories: An Example from the Automotive Industry. IFAC-Pap. 2016, 49, 97–102. https://doi.org/10.1016/j.ifacol.2016.12.168.
- Qi, Q.; Tao, F. Digital Twin and Big Data Towards Smart Manufacturing and Industry 4.0: 360 Degree Comparison. IEEE Access 2018, 6, 3585–3593. https://doi.org/10.1109/ACCESS.2018.2793265.
- Tao, F.; Cheng, J.; Qi, Q.; Zhang, M.; Zhang, H.; Sui, F. Digital twin-driven product design, manufacturing and service with big data. Int. J. Adv. Manuf. Technol. 2018, 94, 3563–3576. https://doi.org/10.1007/s00170-017-0233-1.
- Tao, F.; Zhang, M.; Liu, Y.; Nee, A.Y.C. Digital twin driven prognostics and health management for complex equipment. CIRP Ann. 2018, 67, 169–172. https://doi.org/10.1016/j.cirp.2018.04.055.
- Liu, M.; Fang, S.; Dong, H.; Xu, C. Review of digital twin about concepts, technologies, and industrial applications. J. Manuf. Syst. 2021, 58, 346–361. https://doi.org/10.1016/j.jmsy.2020.06.017.
- PricewaterhouseCoopers. Annual Manufacturing Report 2020. 2020. Available online: https://www.pwc.co.uk/industries/manufacturing/insights/annual-manufacturing-report.html (accessed on 2 August 2022).
- Lo, C.K.; Chen, C.H.; Zhong, R.Y. A review of digital twin in product design and development. Adv. Eng. Inform. 2021, 48, 101297. https://doi.org/10.1016/j.aei.2021.101297.
- Grieves, M.; Vickers, J. Digital Twin: Mitigating Unpredictable, Undesirable Emergent Behavior in Complex Systems. In Transdisciplinary Perspectives on Complex Systems: New Findings and Approaches; Kahlen, F.-J., Flumerfelt, S., Alves, A., Eds.; Springer International Publishing: Cham, Switzerland, 2017; pp. 85–113. https://doi.org/10.1007/978-3-319-38756-7_4.
- Xie, G.; Yang, K.; Xu, C.; Li, R.; Hu, S. Digital Twinning Based Adaptive Development Environment for Automotive Cyber-Physical Systems. IEEE Trans. Ind. Inform. 2022, 18, 1387–1396. https://doi.org/10.1109/TII.2021.3064364.
- Deng, S.; Ling, L.; Zhang, C.; Li, C.; Zeng, T.; Zhang, K.; Guo, G. A systematic review on the current research of digital twin in automotive application. Internet Things Cyber-Phys. Syst. 2023, 3, 180–191. https://doi.org/10.1016/j.iotcps.2023.04.004.
- Söderberg, R.; Wärmefjord, K.; Carlson, J.S.; Lindkvist, L. Toward a Digital Twin for real-time geometry assurance in individualized production. CIRP Ann. 2017, 66, 137–140. https://doi.org/10.1016/j.cirp.2017.04.038.
- Botín-Sanabria, D.M.; Mihaita, A.-S.; Peimbert-García, R.E.; Ramírez-Moreno, M.A.; Ramírez-Mendoza, R.A.; Lozoya-Santos, J.D.J. Digital Twin Technology Challenges and Applications: A Comprehensive Review. Remote Sens. 2022, 14, 1335. https://doi.org/10.3390/rs14061335.
- Qi, Q.; Tao, F.; Hu, T.; Anwer, N.; Liu, A.; Wei, Y.; Nee, A.Y. Enabling technologies and tools for digital twin. J. Manuf. Syst. 2021, 58, 3–21. https://doi.org/10.1016/j.jmsy.2019.10.001.
- El Fakir, O.; Wang, L.; Balint, D.; Dear, J.P.; Lin, J.; Dean, T.A. Numerical study of the solution heat treatment, forming, and in-die quenching (HFQ) process on AA5754. Int. J. Mach. Tools Manuf. 2014, 87, 39–48. https://doi.org/10.1016/j.ijmachtools.2014.07.008.
- Wang, K.; Kopec, M.; Chang, S.; Qu, B.; Liu, J.; Politis, D.J.; Liu, G. Enhanced formability and forming efficiency for two-phase titanium alloys by Fast light Alloys Stamping Technology (FAST). Mater. Des. 2020, 194, 108948. https://doi.org/10.1016/j.matdes.2020.108948.
- Li, H.; Li, B.; Liu, G.; Wen, X.; Wang, H.; Wang, X.; Yang, W. A detection and configuration method for welding completeness in the automotive body-in-white panel based on digital twin. Sci. Rep. 2022, 12, 7929. https://doi.org/10.1038/s41598-022-11440-0.
- Zheng, K.; Dong, Y.; Zheng, J.H.; Foster, A.; Lin, J.; Dong, H.; Dean, T.A. The effect of hot form quench (HFQ®) conditions on precipitation and mechanical properties of aluminium alloys. Mater. Sci. Eng. A 2019, 761, 138017. https://doi.org/10.1016/j.msea.2019.06.027.
- Zhang, Q.; Luan, X.; Dhawan, S.; Politis, D.J.; Du, Q.; Fu, M.W.; Wang, L. Development of the post-form strength prediction model for a high-strength 6xxx aluminium alloy with pre-existing precipitates and residual dislocations. Int. J. Plast. 2019, 119, 230–248. https://doi.org/10.1016/j.ijplas.2019.03.013.
- Sun, Y.; Wang, K.; Politis, D.J.; Chen, G.; Wang, L. An experimental investigation on the ductility and post-form strength of a martensitic steel in a novel warm stamping process. J. Mater. Process. Technol. 2020, 275, 116387. https://doi.org/10.1016/j.jmatprotec.2019.116387.
- Zhou, C.; Zhang, F.; Wei, B.; Lin, Y.; He, K.; Du, R. Digital twin–based stamping system for incremental bending. Int. J. Adv. Manuf. Technol. 2021, 116, 389–401. https://doi.org/10.1007/s00170-021-07422-7.
- Wang, Q.; Jiao, W.; Zhang, Y. Deep learning-empowered digital twin for visualized weld joint growth monitoring and penetration control. J. Manuf. Syst. 2020, 57, 429–439. https://doi.org/10.1016/j.jmsy.2020.10.002.
- Liu, C.; Le Roux, L.; Körner, C.; Tabaste, O.; Lacan, F.; Bigot, S. Digital Twin-enabled Collaborative Data Management for Metal Additive Manufacturing Systems. J. Manuf. Syst. 2022, 62, 857–874. https://doi.org/10.1016/j.jmsy.2020.05.010.
- Chantzis, D.; Tracy, M.; Liu, H.; Politis, D.J.; Fu, M.W.; Wang, L. Design Optimization of Hot Stamping Tooling produced by Additive Manufacturing. Addit. Manuf. 2023, 74, 103728. https://doi.org/10.1016/j.addma.2023.103728.
- Phua, A.; Davies, C.H.J.; Delaney, G.W. A digital twin hierarchy for metal additive manufacturing. Comput. Ind. 2022, 140, 103667. https://doi.org/10.1016/j.compind.2022.103667.
- Gunasegaram, D.R.; Murphy, A.B.; Barnard, A.; DebRoy, T.; Matthews, M.J.; Ladani, L.; Gu, D. Towards developing multiscale-multiphysics models and their surrogates for digital twins of metal additive manufacturing. Addit. Manuf. 2021, 46, 102089. https://doi.org/10.1016/j.addma.2021.102089.
- Dilberoglu, U.M.; Gharehpapagh, B.; Yaman, U.; Dolen, M. The Role of Additive Manufacturing in the Era of Industry 4.0. Procedia Manuf. 2017, 11, 545–554. https://doi.org/10.1016/j.promfg.2017.07.148.
- Masinelli, G.; Shevchik, S.A.; Pandiyan, V.; Quang-Le, T.; Wasmer, K. Artificial Intelligence for Monitoring and Control of Metal Additive Manufacturing. In Industrializing Additive Manufacturing; Meboldt, M., Klahn, C., Eds.; Springer International Publishing: Cham, Switzerland, 2021; pp. 205–220. https://doi.org/10.1007/978-3-030-54334-1_15.
- Elambasseril, J.; Brandt, M. Artificial intelligence: Way forward to empower metal additive manufacturing product development–an overview. Mater. Today Proc. 2022, 58, 61–465. https://doi.org/10.1016/j.matpr.2022.02.485.
- Wang, C.; Tan, X.P.; Tor, S.B.; Lim, C.S. Machine learning in additive manufacturing: State-of-the-art and perspectives. Addit. Manuf. 2020, 36, 101538. https://doi.org/10.1016/j.addma.2020.101538.
- Rathore, M.M.; Shah, S.A.; Shukla, D.; Bentafat, E.; Bakiras, S. The Role of AI, Machine Learning, and Big Data in Digital Twinning: A Systematic Literature Review, Challenges, and Opportunities. IEEE Access 2021, 9, 32030–32052. https://doi.org/10.1109/ACCESS.2021.3060863.
- Vachálek, J.; Bartalský, L.; Rovný, O.; Šišmišová, D.; Morháč, M.; Lokšík, M. The digital twin of an industrial production line within the industry 4.0 concept. In Proceedings of the 2017 21st International Conference on Process Control (PC), Strbske Pleso, Slovakia, 6–9 June 2017; pp. 258–262. https://doi.org/10.1109/PC.2017.7976223.
- Bhatti, G.; Mohan, H.; Singh, R.R. Towards the future of smart electric vehicles: Digital twin technology. Renew. Sustain. Energy Rev. 2021, 141, 110801, May. https://doi.org/10.1016/j.rser.2021.110801.
- Son, Y.H.; Park, K.T.; Lee, D.; Jeon, S.W.; Noh, S.D. Digital twin–based cyber-physical system for automotive body production lines. Int. J. Adv. Manuf. Technol. 2021, 115, 291–310. https://doi.org/10.1007/s00170-021-07183-3.
- Mendi, A.F. A Digital Twin Case Study on Automotive Production Line. Sensors 2022, 22, 6963. https://doi.org/10.3390/s22186963.
- Zhang, Q.; Shen, S.; Li, H.; Cao, W.; Tang, W.; Jiang, J.; Liu, S. Digital twin-driven intelligent production line for automotive MEMS pressure sensors. Adv. Eng. Inform. 2022, 54, 101779. https://doi.org/10.1016/j.aei.2022.101779.
- Xu, Y.; Sun, Y.; Liu, X.; Zheng, Y. A Digital-Twin-Assisted Fault Diagnosis Using Deep Transfer Learning. IEEE Access 2019, 7, 19990–19999. https://doi.org/10.1109/ACCESS.2018.2890566.
- Biesinger, F.; Meike, D.; Kraß, B.; Weyrich, M. A Case Study for a Digital Twin of Body-in-White Production Systems General Concept for Automated Updating of Planning Projects in the Digital Factory. In Proceedings of the 2018 IEEE 23rd International Conference on Emerging Technologies and Factory Automation (ETFA), Turin, Italy, 4–7 September 2018; pp. 19–26. https://doi.org/10.1109/ETFA.2018.8502467.
- Biesinger, F.; Kraß, B.; Weyrich, M. A Survey on the Necessity for a Digital Twin of Production in the Automotive Industry. In Proceedings of the 2019 23rd International Conference on Mechatronics Technology (ICMT), Salerno, Italy, 23–26 October 2019; pp. 1–8. https://doi.org/10.1109/ICMECT.2019.8932144.
- Voulgaridis, K.; Lagkas, T.; Angelopoulos, C.M.; Boulogeorgos, A.-A.A.; Argyriou, V.; Sarigiannidis, P. Digital product passports as enablers of digital circular economy: A framework based on technological perspective. Telecommun. Syst. 2024, 85, 699–715. https://doi.org/10.1007/s11235-024-01104-x.
- Proposal for a Regulation of the European Parliament and of the Council Establishing a Framework for Setting Ecodesign Requirements for Sustainable Products and Repealing Directive 2009/125/EC; European Commission: Brussels, Belgium, 2022. Available online: https://scholar.google.com/scholar_lookup?title=Proposal+for+a+Regulation+of+the+European+Parliament+and+of+the+Council+Establishing+a+Framework+for+Setting+Ecodesign+Requirements+for+Sustainable+Products+and+Repealing+Directive+2009125EC&publication_year=2022&author=European+Commission&inst=4887157489608331491 (accessed on 27 February 2024).
- Berger, K.; Schöggl, J.-P.; Baumgartner, R.J. Concept of a digital product passport for an electric vehicle battery. Presented at the Resource Efficient Vehicles Conference, Graz, Austria, 14–16 June 2021. Available online: http://axaco.s3.amazonaws.com/uploads/2021/05/17/uqYgqc6e/rev2021-048.pdf (accessed on 27 February 2024).
- Ruah, C.; Simeone, O.; Al-Hashimi, B.M. A Bayesian Framework for Digital Twin-Based Control, Monitoring, and Data Collection in Wireless Systems. IEEE J. Sel. Areas Commun. 2023, 41, 3146–3160. https://doi.org/10.1109/JSAC.2023.3310093.
- Dogan, A.; Birant, D. Machine learning and data mining in manufacturing. Expert Syst. Appl. 2021, 166, 114060. https://doi.org/10.1016/j.eswa.2020.114060.
- Deloitte Survey on AI Adoption in Manufacturing. 2020. Available online: https://www2.deloitte.com/cn/en/pages/consumer-industrial-products/articles/ai-manufacturing-application-survey.html (accessed on 13 December 2022).
- Liu, S.; Xia, Y.; Liu, Y.; Shi, Z.; Yu, H.; Li, Z.; Lin, J. Tool path planning of consecutive free-form sheet metal stamping with deep learning. J. Mater. Process. Technol. 2022, 303, 117530. https://doi.org/10.1016/j.jmatprotec.2022.117530.
- Wilkinson, M.D.; Dumontier, M.; Jan Aalbersberg, I.J.; Appleton, G.; Axton, M.; Baak, A.; Blomberg, N.; Boiten, J.-W.; da Silva Santos, L.B.; Bourne, P.E.; et al. The FAIR Guiding Principles for scientific data management and stewardship. Scientific Data 2016, 3, 160018. https://doi.org/10.1038/sdata.2016.18.
- Zhou, D.; Yuan, X.; Gao, H.; Wang, A.; Liu, J.; El Fakir, O.; Lin, J. Knowledge Based Cloud FE Simulation of Sheet Metal Forming Processes. J. Vis. Exp. 2016, 118, e53957. https://doi.org/10.3791/53957.
- Barker, M.; Chue Hong, N.P.; Katz, D.S.; Lamprecht, A.L.; Martinez-Ortiz, C.; Psomopoulos, F.; Honeyman, T. Introducing the FAIR Principles for research software. Sci. Data 2022, 9, 622. https://doi.org/10.1038/s41597-022-01710-x.
- Wang, A.; Liu, J.; Gao, H.; Wang, L.-L.; Masen, M. Hot stamping of AA6082 tailor welded blanks: Experiments and knowledge-based cloud–finite element (KBC-FE) simulation. J. Mater. Process. Technol. 2017, 250, 228–238. https://doi.org/10.1016/j.jmatprotec.2017.07.025.
- Wang, A. Multi-Objective Finite Element Simulations of a Sheet Metal Forming Process via a Knowledge Based Cloud Simulation Platform; Imperial College London: London, UK, 2018. Available online: http://spiral.imperial.ac.uk/handle/10044/1/86263 (accessed on 23 June 2022).
- Dhawan, S.; El Fakir, O.; Wang, L. An Online Database for Hosting and Executing Numerical Models. U.S. Patent 201911116210.6, 25 February 2020.
- Zhu, M.; Lim, Y.C.; Cai, Z.; Liu, X.; Dhawan, S.; Liu, J.; Politis, D.J. Cloud FEA of hot stamping processes using a software agnostic platform. Int. J. Adv. Manuf. Technol. 2021, 112, 3445–3458. https://doi.org/10.1007/s00170-020-06533-x.
- Dhawan, S. Development of a Cloud FEA Platform for Advanced FE Simulations of Metal Forming Processes; Imperial College London: London, UK, 2022.
- Yang, X.; Liu, H.; Zhang, L.; Hu, Y.; Politis, D.J.; Gharbi, M.M.; Wang, L. Interactive mechanism and friction modelling of transient tribological phenomena in metal forming processes: A review. Friction 2024, 12, 375–395. https://doi.org/10.1007/s40544-023-0751-9.
- Kouhizadeh, M.; Zhu, Q.; Sarkis, J. Blockchain and the circular economy: Potential tensions and critical reflections from practice. Prod. Plan. Control 2020, 31, 950–966. https://doi.org/10.1080/09537287.2019.1695925.
- Kumar, N.M.; Chopra, S.S. Leveraging Blockchain and Smart Contract Technologies to Overcome Circular Economy Implementation Challenges. Sustainability 2022, 14, 9492. https://doi.org/10.3390/su14159492.
- Adisorn, T.; Tholen, L.; Götz, T. Towards a Digital Product Passport Fit for Contributing to a Circular Economy. Energies 2021, 14, 2289. https://doi.org/10.3390/en14082289.
- Yang, X.; Liu, H.; Dhawan, S.; Politis, D.J.; Zhang, J.; Dini, D.; Wang, L. Digitally-enhanced lubricant evaluation scheme for hot stamping applications. Nat. Commun. 2022, 13, 5748. https://doi.org/10.1038/s41467-022-33532-1.
- Mia, M.; Zhang, L.; Anwar, S.; Liu, H. Development of digital characteristics of machining based on physics-guided data. J. Manuf. Syst. 2023, 71, 438–450. https://doi.org/10.1016/j.jmsy.2023.09.014.
- Liu, H.; Liu, X.; Yang, X.; Politis, D.J.; Zheng, Y.; Dhawan, S.; Wang, L. Mapping the hot stamping process through developing distinctive digital characteristics. Comput. Ind. 2024, 161, 104121. https://doi.org/10.1016/j.compind.2024.104121.
- Liu, H.; Dhawan, S.; Yang, X.; Politis, D.J.; Weill, M.; Zheng, Y.; Wang, L. Genetic exploration” of metal forming processes through information absent and fragmental data processing. J. Manuf. Syst. 2025, 79, 286–300. https://doi.org/10.1016/j.jmsy.2025.01.014.
- Liu, H.; Yang, X.; Weill, M.; Li, S.; Wu, V.; Politis, D.J.; Wang, L. Unlocking inherent values of manufacturing metadata through digital characteristics (DC) alignment. Comput. Ind. 2024, 163, 104148. https://doi.org/10.1016/j.compind.2024.104148.
- Liu, H.; Yang, X.; Politis, D.J.; Shi, H.; Wang, L. Evaluation framework of digital characteristics (DC) enhanced lubricant: Consideration of essential geometric features for hot-stamped components. J. Manuf. Syst. 2024, 75, 150–162. https://doi.org/10.1016/j.jmsy.2024.06.008.
- Liu, H.; Wu, V.; Weill, M.; Li, S.; Yang, X.; Politis, D.J.; Wang, L. Developing physics-informed filters to align unattributed fragmental manufacturing data against a digital characteristics space (DCS). J. Manuf. Syst. 2024, 77, 18–25. https://doi.org/10.1016/j.jmsy.2024.09.002.
- Liu, H.; Yang, X.; Politis, D.; Shi, H.; Wang, L. An evaluation scheme incorporating digital characteristics for transient tribological behaviours under complex loading conditions for the hot stamping process. Comput. Ind. 2025, 167, 104270. https://doi.org/10.1016/j.compind.2025.104270.
- Javaid, M.; Haleem, A.; Suman, R. Digital Twin applications toward Industry 4.0: A Review. Cogn. Robot. 2023, 3, 71–92. https://doi.org/10.1016/j.cogr.2023.04.003.